by Sue Denim
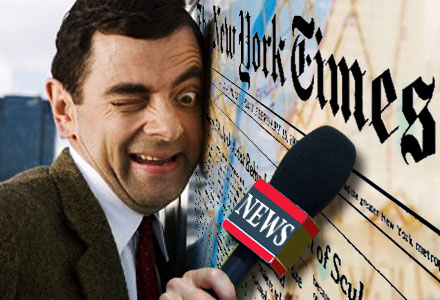
A source of frustration for many of us is how journalists don’t seem to be learning lessons about academic models. It’s as though their need to report on anything new makes them extremely vulnerable to trusting the output of discredited processes and people.
Take this piece in UnHerd by Tom Chivers called “What’s the point of contact tracing?” It’s about a paper entitled “Modelling the health and economic impacts of Population-wide Testing, contact Tracing and Isolation (PTTI) strategies for COVID-19 in the UK“. It starts with a brief hint that maybe epidemiology models can be problematic (my emphases in bold):
Well, one interesting paper just published by researchers at University College London looks at the impact contact tracing will have on the economy relative to lockdown …
Some caveats: modelling is inherently uncertain. Manheim [an author] says that the model is robust — the headline outcomes remain broadly consistent even if the assumptions and inputs are tweaked — but it’s just one paper, and so far not peer-reviewed.
Note how the academic’s opinion of his own work is given as evidence it’s correct, as if this provides any insight. This paper has a whopping 26 authors, yet they apparently couldn’t wait for peer review before sending it to journalists. Let’s do a quick review for them.
The introduction says this:
Mathematical models can be used to predict COVID-19 epidemic trends following relaxation of lockdown.
It provides a citation for this claim, the first in the paper: Professor Ferguson’s discredited Report 9. ICL’s model in reality proves the opposite – it couldn’t predict COVID-19 epidemic trends as evidenced by the results when applied to Sweden. And as is by now well documented, this work has severe and retraction-worthy methodological errors that make it unsuitable for citation. Given the media coverage of the many flaws in Report 9, it’s unlikely the authors of this new paper didn’t know that.
And the paper goes downhill from there. It states:
We assume an infection fatality rate (IFR) of 1.0%
No source for this number is provided. There are many studies showing a far lower estimated IFR, more in the range of 0.1% – an order of magnitude difference. The US CDC has dropped its best estimate of the IFR to <0.3%. Assuming an IFR of 1.0% requires significant discussion and evidence to contradict all those other sources, but none is provided.
Chivers says “UnHerd has spoken to various people who think it’s lower than that” but otherwise accepts this exceptionally high value as a given. The story wouldn’t work if he didn’t.
The paper goes on to say (my emphasis):
Recent estimates suggest that only 6.8% of the population… had been infected… this level of presumed immunity is a long way from the roughly 60% required for herd immunity without “overshoot”…. Consequently… without implementing effective provision of testing, contact tracing, and isolation, in conjunction with other measures, the UK may be at risk of either spending two thirds to three quarters of time locked down, or experiencing an uncontrolled epidemic with between 250,000 and 550,000 deaths.
The authors assume the 60% figure although the epidemic ended in many places without that level being achieved, implying it cannot possibly be correct. At least one paper has explored whether this is caused by people having some pre-existing immunity from common cold infections. They also double down on by now bizarre and absurd predictions of an “uncontrolled epidemic” with half a million deaths, in spite of the fact that countries and states that hardly locked down at all have seen no difference in how the epidemic proceeded.
Still, it’s not all bad. Their software is at least published on GitHub along with the paper this time. It’s written in Python, which is harder to screw up than C. They also helpfully document their assumptions. In prior rounds some people have raised the criticism that errors introduced via bugs are likely to be small relative to assumption errors. For programs written in C this isn’t really the case (as I explain in the appendix of this article). But let’s make an assumption of our own here and assume there are no bugs in this new model. Let’s instead look at their assumptions.
We start with a meta-scientific assumption. The README contains a comment indicative of the catastrophic mentality epidemiologists have developed:
we believe in checking models against each other, as it’s the best way to understand which models work best in what circumstances.
No. The best way to understand which models work best is to compare them to reality, not to other models. This circular definition of success has been presented by Imperial College as well. It seems the field of epidemiology has completely lost its grip on the scientific method.
In the documentation the authors provide a variety of arguments for why their research isn’t invalid despite making assumptions they know are wrong. But often the explanations boil down to “just because” (my emphasis):
[We assume] The outbreak or epidemic is homogeneous in space and time. In reality, the UK is undergoing several COVID-19 outbreaks… In general, a model of a single large outbreak cannot be used to reproduce the dynamics of smaller outbreaks separated in time… This model does not represent those different, coupled outbreaks, it represents a single outbreak.Justification: this is a simplifying assumption. In the case of COVID-19, the major outbreaks in the most populous cities are separated in time by only a couple of generations. We argue that this is close enough that, to a first approximation, the differences can be disregarded and valid insights gained by considering the ensemble as one large outbreak
Although they say they “argue” this assumption doesn’t matter, no actual argument is provided in the documentation. They just assert it’s all fine. The paper doesn’t appear to contain such an argument either.
As they admit, the real reason for this assumption is just to make the coding easier.
[We assume] The population is homogeneous and each individual behaves in the same way and is affected in the same way by the virus. This is manifestly untrue of the real world. Justification: this is another simplifying assumption.
Shouldn’t they fix these before making predictions?
Last one (my emphasis):
Face coverings were assumed to reduce 𝛽 by 30%. This is based on an estimated 60% effectiveness of face coverings in reducing transmission and a conservative assumption that they would only be worn 50% of the time, i.e. for 50% of the contacts occurring in the modelled scenario trajectories.
These two claims sound like they’re based on real observations. Of the papers cited, neither appears to actually contain these claims. The first bolded sentence cites this paper which is the output of yet another model. The second sentence cites this response to criticism, which doesn’t appear to talk about how long people wear masks for at all. It’s unclear why they think it justifies this “conservative” assumption that so neatly happens to be exactly 50% – not more or less.
Although this paper hasn’t been peer reviewed it’s unlikely the peer review process in epidemiology would filter this sort of problem out. Papers in this field appear to be worse than useless – not only do they routinely describe a fantasy world the authors know isn’t real, but nobody seems to care. Circular reasoning runs rampant and yet journalists like Chivers – who is more scientifically literate than most – still present the resultant “findings” to the population, knowing that readers won’t call them out on it.
It’s time readers demand journalists push the mute button on these kinds of papers and the modellers who write them. If academics want to be taken seriously in future, they should start creating public databases of past events and matching new outbreaks to them instead of trying to simulate whole societies. Empirical observation of the past can then be applied to estimate the future. Although this won’t require fancy modelling skills and may yield only a few papers, the results would be far more useful.
To join in with the discussion please make a donation to The Daily Sceptic.
Profanity and abuse will be removed and may lead to a permanent ban.