Since 2016 automated Twitter accounts have been blamed for Donald Trump and Brexit (many times), Brazilian politics, Venezuelan politics, skepticism of climatology, cannabis misinformation, anti-immigration sentiment, vaping, and, inevitably, distrust of COVID vaccines. News articles about bots are backed by a surprisingly large amount of academic research. Google Scholar alone indexes nearly 10,000 papers on the topic. Some of these papers received widespread coverage:
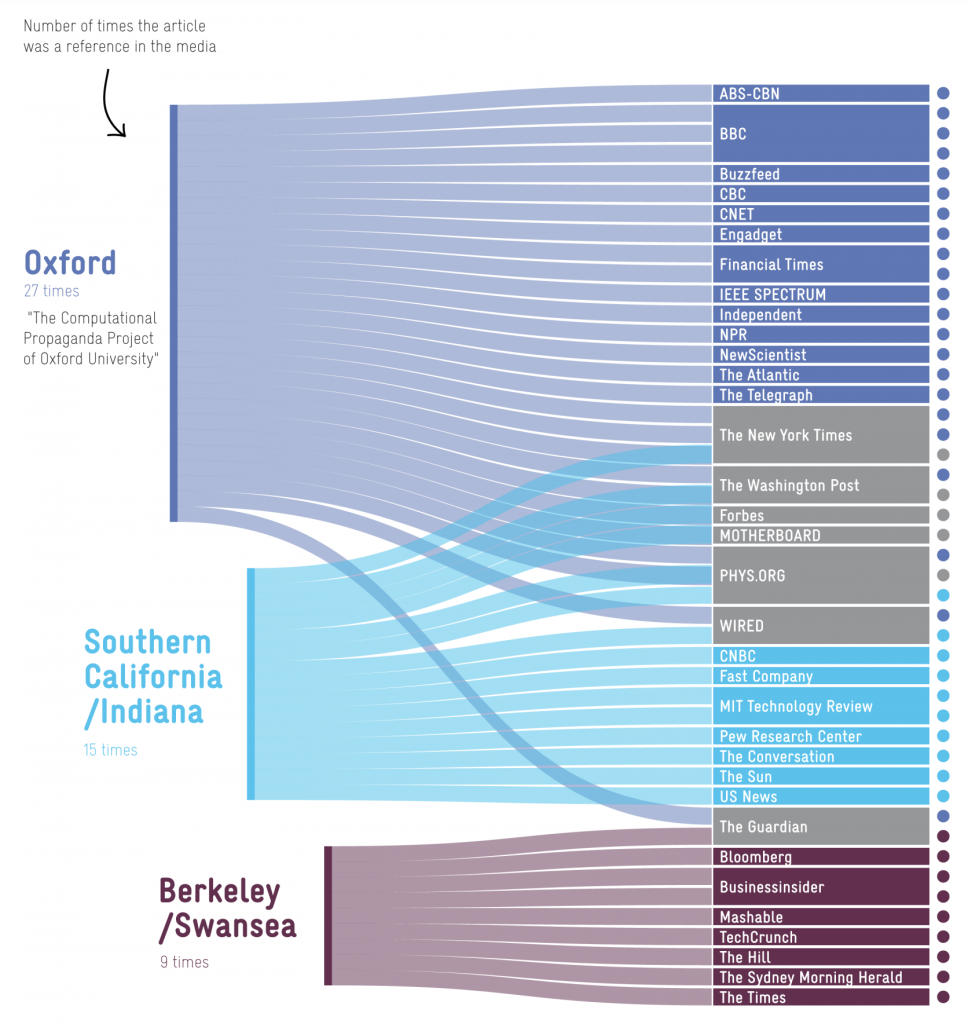
Unfortunately there’s a problem with this narrative: it is itself misinformation. Bizarrely and ironically, universities are propagating an untrue conspiracy theory while simultaneously claiming to be defending the world from the very same.
The visualization above comes from “The Rise and Fall of Social Bot Research” (also available in talk form). It was quietly uploaded to a preprint server in March by Gallwitz and Kreil, two German investigators, and has received little attention since. Yet their work completely destroys the academic field of bot research to such an extreme extent that it’s possible there are no true scientific papers on the topic at all.
The authors identify a simple problem that crops up in every study they looked at. Unable to directly detect bots because they don’t work for Twitter, academics come up with proxy signals that are asserted to imply automation but which actually don’t. For example, Oxford’s Computational Propaganda Project – responsible for the first paper in the diagram above – defined a bot as any account that tweets more than 50 times per day. That’s a lot of tweeting but easily achieved by heavy users, like the famous journalist Glenn Greenwald, the slightly less famous member of German Parliament Johannes Kahrs – who has in the past managed to rack up an astounding 300 tweets per day – or indeed Donald Trump, who exceeded this threshold on six different days during 2020. Bot papers typically don’t provide examples of the bot accounts they claimed to identify, but in this case four were presented. Of those, three were trivially identifiable as (legitimate) bots because they actually said they were bots in their account metadata, and one was an apparently human account claimed to be a bot with no evidence. On this basis the authors generated 27 news stories and 323 citations, although the paper was never peer reviewed.
In 2017 I investigated the Berkley/Swansea paper and found that it was doing something very similar, but using an even laxer definition. Any account that regularly tweeted more than five times after midnight from a smartphone was classed as a bot. Obviously, this is not a valid way to detect automation. Despite being built on nonsensical premises, invalid modelling, mis-characterisations of its own data and once again not being peer reviewed, the authors were able to successfully influence the British Parliament. Damian Collins, the Tory MP who chaired the DCMS Select Committee at the time, said: “This is the most significant evidence yet of interference by Russian-backed social media accounts around the Brexit referendum. The content published and promoted by these accounts is clearly designed to increase tensions throughout the country and undermine our democratic process. I fear that this may well be just the tip of the iceberg.”
But since 2019 the vast majority of papers about social bots rely on a machine learning model called ‘Botometer’. The Botometer is available online and claims to measure the probability of any Twitter account being a bot. Created by a pair of academics in the USA, it has been cited nearly 700 times and generates a continual stream of news stories. The model is frequently described as a “state of the art bot detection method” with “95% accuracy”.
That claim is false. The Botometer’s false positive rate is so high it is practically a random number generator. A simple demonstration of the problem was the distribution of scores given to verified members of U.S. Congress:
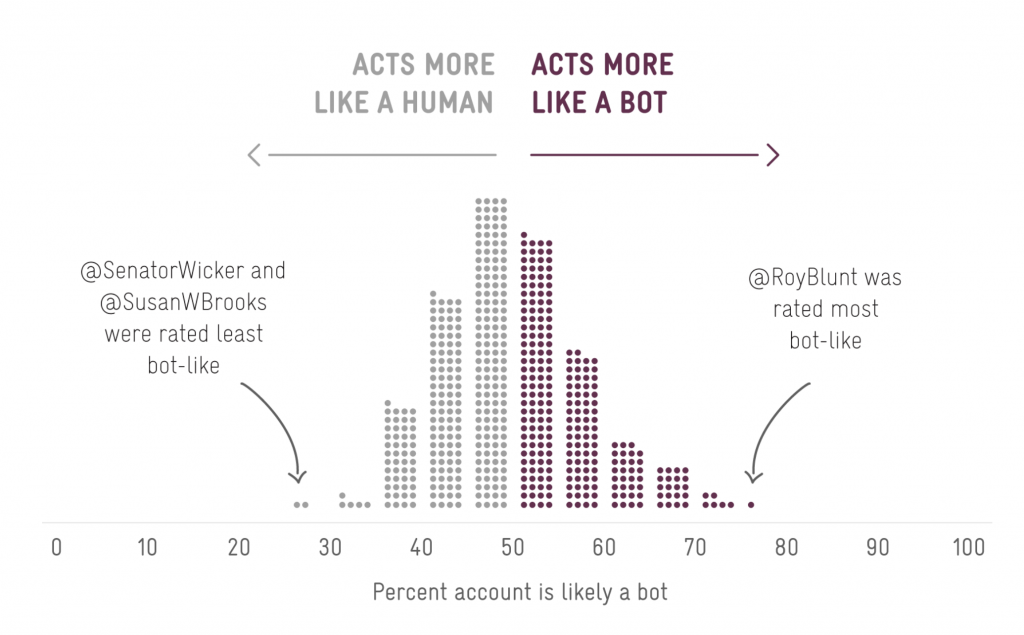
In experiments run by Gallwitz & Kreil, nearly half of Congress were classified as more likely to be bots than human, along with 12% of Nobel Prize laureates, 17% of Reuters journalists, 21.9% of the staff members of U.N. Women and – inevitably – U.S. President Joe Biden.
But detecting the false positive problem did not require compiling lists of verified humans. One study that claimed to identify around 190,000 bots included the following accounts in its set:
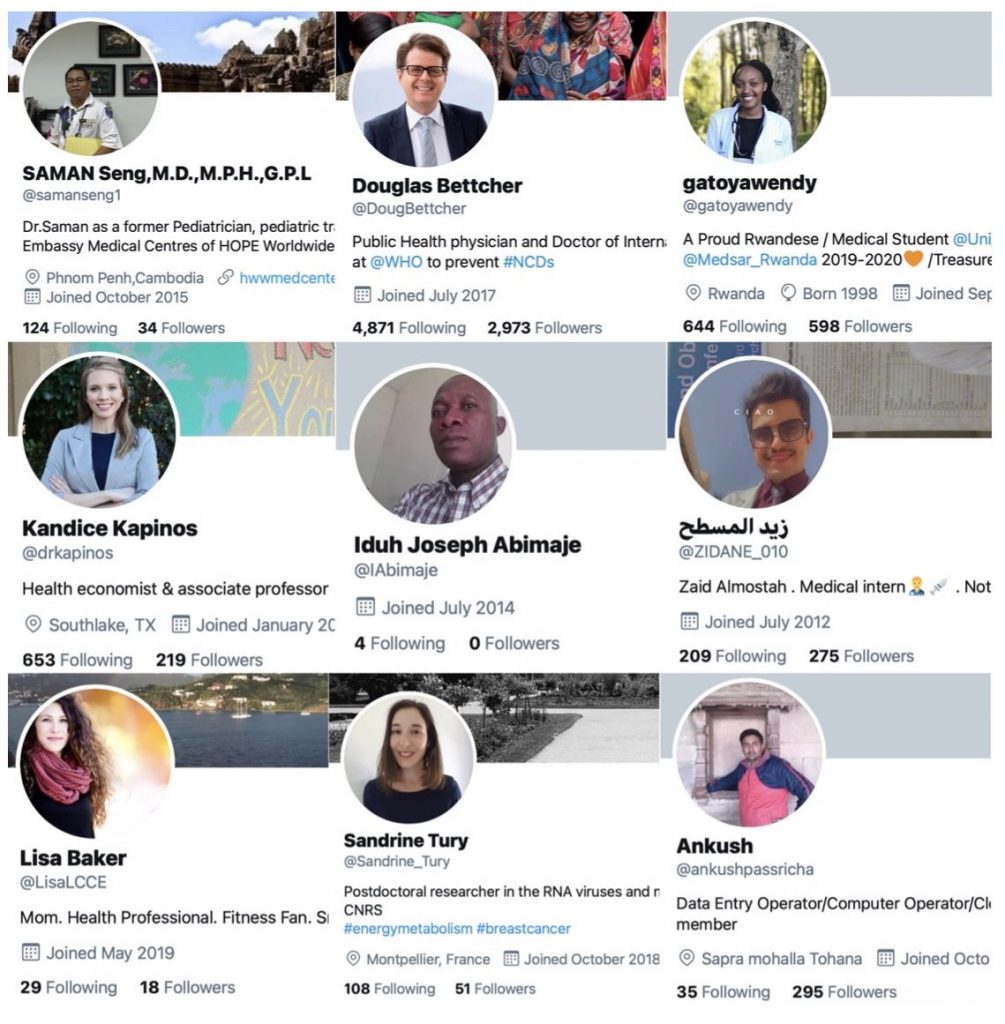
The developers of the Botometer know it doesn’t work. After the embarrassing U.S. Congress data was published, an appropriate response would have been retraction of their paper. But that would have implied that all the papers that relied upon it should also be retracted. Instead they hard-coded the model to know that Congress are human and then went on the attack, describing their critics as “academic trolls”:
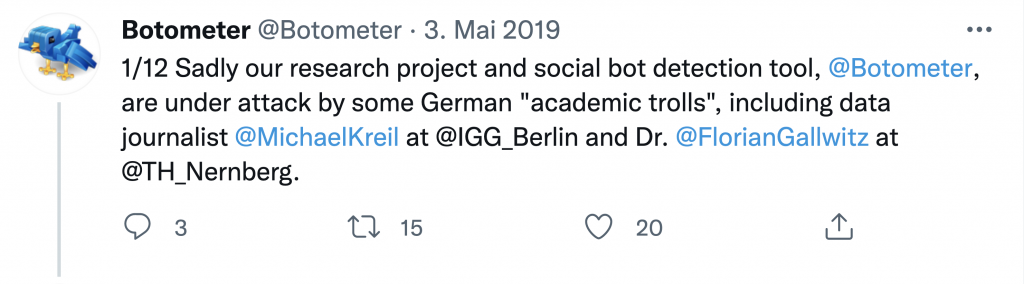
Root cause analysis
This story is a specific instance of a general problem that crops up frequently in bad science. Academics decide a question is important and needs to be investigated, but they don’t have sufficiently good data to draw accurate conclusions. Because there are no incentives to recognize that and abandon the line of inquiry, they proceed regardless and make claims that end up being drastically wrong. Anyone from outside the field who points out what’s happening is simply ignored, or attacked as “not an expert” and thus inherently illegitimate.
Although no actual expertise is required to spot the problems in this case, I can nonetheless criticize their work with confidence because I actually am an expert in fighting bots. As a senior software engineer at Google I initiated and designed one of their most successful bot detection platforms. Today it checks over a million actions per second for malicious automation across the Google network. A version of it was eventually made available to all websites for free as part of the ReCAPTCHA system, providing an alternative to the distorted word puzzles you may remember from the earlier days of the internet. Those often frustrating puzzles were slowly replaced in recent years by simply clicking a box that says “I’m not a bot”. The latest versions go even further and can detect bots whilst remaining entirely invisible.
Exactly how this platform works is a Google trade secret, but when spammers discuss ideas for beating it they are well aware that it doesn’t use the sort of techniques academics do. Despite the frequent claim that Botometer is “state of the art”, in reality it is primitive. Genuinely state-of-the-art bot detectors use a correct definition of bot based on how actions are being performed. Spammers are forced to execute polymorphic encrypted programs that detect signs of automation at the protocol and API level. It’s a battle between programmers, and how it works wouldn’t be easily explainable to social scientists.
Spam fighters at Twitter have an equally low opinion of this research. They noted in 2020 that tools like Botometer use “an extremely limited approach” and “do not account for common Twitter use cases”. “Binary judgments of who’s a “bot or not” have real potential to poison our public discourse – particularly when they are pushed out through the media …. the narrative on what’s actually going on is increasingly behind the curve.”
Many fields cannot benefit from academic research because academics cannot obain sufficiently good data with which to draw conclusions. Unfortunately, they sometimes have difficulty accepting that. When I ended my 2017 investigation of the Berkeley/Swansea paper by observing that social scientists can’t usefully contribute to fighting bots, an academic posted a comment calling it “a Trumpian statement” and argued that tech firms should release everyone’s private account data to academics, due to their capacity for “more altruistic” insights. Yet their self-proclaimed insights are usually far from altruistic. The ugly truth is that social bot research is primarily a work of ideological propaganda. Many bot papers use the supposed prevalence of non-existent bots to argue for censorship and control of the internet. Too many people disagree with common academic beliefs. If only social media were edited by the most altruistic and insightful members of society, they reason, nobody would ever disagree with them again.
To join in with the discussion please make a donation to The Daily Sceptic.
Profanity and abuse will be removed and may lead to a permanent ban.