As 2022 ends, the yearly death totals are rolled out by various media outlets as a source of concern.
The Times reports 1,000 excess deaths each week as the NHS buckles, with 656,735 U.K. deaths in the last year. Using the pre-Covid five-year average, it notes, “50,000 more people died last year than normal”.
The BBC reported that 9% more people died in 2022 than in 2019. Within hours of the reports of the deaths, they concluded the “data indicates pandemic effects on health and NHS pressures are among the leading explanations”.
No need then for painstaking epidemiology, assessment of confounders and determination of causation as opposed to an association. The BBC has the answer. But does it?
One of its explanations is the “lasting effect of pandemic”. Pointing to a “number of studies that found people are more likely to have heart problems and strokes“.
All three studies cited are retrospective. The first is a review of those admitted to the hospital in 2020 (mean follow-up 140 days). The second is a review from 2020, comparing U.S. individuals in the Veteran Affairs program who were predominately white males with a positive Covid test with matched controls from 2017. The third was much the same, reporting excess risk in the four months after a positive test.
It is hard to infer that these studies inform lasting effects; the data are from 2020 (before vaccines were introduced), in selected populations (e.g., those admitted to hospital), with short-term follow-up (e.g., four months), in individuals infected with different variants to now, which as one of the reviews points out, “it is possible that the epidemiology of cardiovascular manifestations in COVID-19 might also change over time”, and finally biases due to the study type and selection of controls.
Underlying the BBC statements is the failure to separate the effects of SARS-CoV-2 infection (which the cited studies analyse) from those of restrictions. Why does the BBC conflate two separate possible causes?
But that’s not all. The BBC piece states, “some of this may be contributed to by the fact many people didn’t come in for screenings and non-urgent treatment during the peak of the pandemic”.
This refers to a pre-print paper by Dale et al. on the adverse impact of Covid on cardiovascular disease prevention and management in England, Scotland and Wales. The article reports that 491,203 fewer individuals initiated antihypertensive treatment from March 2020 to the end of May 2021 than expected, and estimated, based on the assumption that none of these received treatments, there could be 13,659 additional cardiovascular disease events (note, not deaths).
We have already reported that the NHS’s data show no decline in prescriptions for any cardiovascular drug since 2019. And we also discussed the Number Needed to Treat (NNT), which for five years of statin treatment in primary prevention is 138 to prevent one death.
The NNT for hypertension treatment, which treatment is for five years to prevent death, is 125. It, therefore, takes time for the effects of the undertreatment of hypertension to become apparent.
So let’s assume that the 500,000 in the Dale et al. paper were never treated. The NNT of 125 means we might expect to see 4,000 extra deaths from hypertensive-related diseases over the next five years.
This does not undermine the importance of blood pressure control. In the U.K., hypertension is the leading modifiable risk factor for heart disease.
The British Heart Foundation estimates 15 million adults have high blood pressure, of which roughly half are not receiving adequate treatment – a third (five million) are undiagnosed.
Furthermore, your risk of death is not just related to the identification of hypertension. Your age, the level of blood pressure and comorbidities make a substantial difference to the risk of death. None of this is accounted for in the retrospective analyses that dominate current research outputs.
The media cycle is designed to produce headlines: find the highest excess estimate and then find some reasons to explain it – job done. Yet, we previously showed estimates of non-Covid excess mortality using historic averages lead to 50-100% higher estimates than methods incorporating trends.
Asking for opinions only fuels speculation about what might be causing the excess. The days of ‘what we think…’ have to end. Evidence-based medicine is hard; it requires high-quality, detailed epidemiological studies. For example, it took a prospective study by Richard Doll to determine the effect of smoking on doctors – one of the largest risk factors for cardiovascular disease death – that followed-up participants for 50 years.
In August, we listed eight non-mutually exclusive causes that require investigating. We’re not sorry for repeating this, but we should test all of these before drawing conclusions.
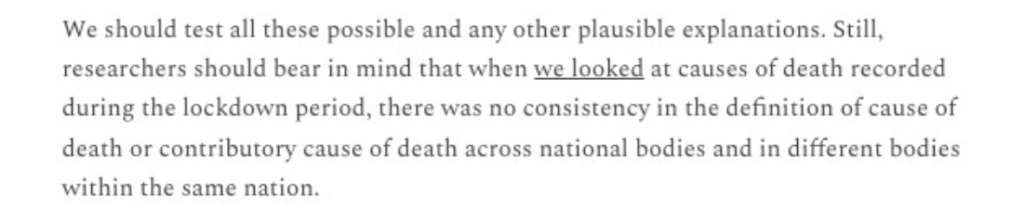
Ascertainment of causation requires serious work, not inferences from studies taken out of their context or headline bait.
Dr. Carl Heneghan is the Oxford Professor of Evidence Based Medicine and Dr. Tom Jefferson is an epidemiologist based in Rome who works with Professor Heneghan on the Cochrane Collaboration. This article was first published on their Substack blog, Trust The Evidence, which you can subscribe to here.
To join in with the discussion please make a donation to The Daily Sceptic.
Profanity and abuse will be removed and may lead to a permanent ban.