Out of the Darkness and Into the Murk
This article takes a look at the quality of the software behind Professor Neil Ferguson of Imperial College London’s infamous “Report 9“. My colleague Derek Winton has already analysed the software methods; I will consider the quality of the development process.
Firstly, you’ll want to inspect my bona fides. I have an MSc in engineering and a lifetime in engineering and IT, most of which has been spent in software and systems testing and is now mainly in data architecture. I have worked as a freelance consultant in about 40 companies including banks, manufacturers and the ONS. The engineer in me really doesn’t like sloppiness or amateurism in software.
We’re going to look at the code that can be found here. This is a public repository containing all the code needed to re-run Prof. Ferguson’s notorious simulations – feel free to download it all and try it yourself. The code will build and run on Windows or Ubuntu Linux and may run on other POSIX-compliant platforms. Endless fun for all the family.
The repository is hosted (stored and managed) in GitHub, which is a widely-used professional tool, probably the world’s most widely-used tool for this purpose. The code and supporting documentation exist in multiple versions so that a full history of development is preserved. The runnable code is built directly from this repository so a very tight control is exercised over the delivered program. So far, so very good industrial practice. The code itself has been investigated several times, so I’ll ignore that and demonstrate some interesting aspects of its metadata.
Firstly, the repository was created on March 22nd 2020, one day before the first – disastrous – lockdown in England was applied. There was a considerable amount of development activity from mid-April until mid-July (see below) and then a decreasing level of activity until by the end of December 2020 development had practically ceased. In February 2021, however, work restarted and peaked in April, falling away again quite quickly. Since then it has been almost entirely quiescent and appears to have been totally silent since July 2021. The repository was substantially complete by mid-June 2020 and almost all activity since then has been in editing existing code.
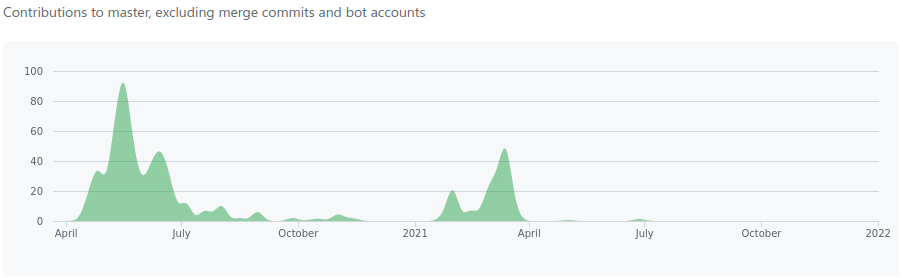
As “Report 9” was published on March 16th 2020, and the criticism of it started almost immediately, it’s very tempting to suggest that the initial period of activity matches the panic to generate something presentable enough to show to the public to support the Government’s initial lockdown. The second campaign of work may be revisions to tune the code to better match the effects of the vaccination programme.
Twenty-two people have contributed to the codebase, including the aforementioned Derek Winton. The lead developer seems to be Wes Hinsley, who is a researcher at ICL. He has an MSc in Computer Engineering and a doctorate in Computing and Earth Sciences, so would appear to be well-qualified to manage the development of something such as this.
This repository is unusual in that it appears to contain every aspect of the software development life cycle – designs and specifications (such as they are), code, tests, test results and defect reports. This is symptomatic of a casual approach to software development management and is often seen in small companies or in unofficial projects in large companies.
However, it does mean that we can gain a good understanding of the real state of the project, which is that it is not as good as I would have hoped it would be for such an influential system. We can read a lot from the “issues” that have been recorded in GitHub.
Issues are things such as test defects, new features, comments and pretty much anything else that people want to be recorded. They are written by engineers for other engineers, so generally are honest and contain good information: these appear to be very much of that ilk. Engineers usually cannot lie; that is why we are rarely ever allowed to meet potential customers before any sale happens. We can trust these comments.
The first issue was added on April 1st 2020 and the last of the 487 so far (two have been deleted) was added on May 6th 2021. Out of the 485 remaining, only two have been labelled as “bugs”, i.e., code faults, which is an unusually low number for this size of a codebase. However, only two are labelled as “enhancements” and in fact a total of 473 (98%) have no label at all, making any analysis practically impossible. This is not good practice.
Only one issue is labelled as “documentation” as there is a branch (folder) specifically for system documentation. The provided documentation looks to be reasonably well laid out but is all marked as WIP – Work in Progress. This means that there is no signed-off design for the software that has been central to the rapid shutdown and slow destruction of our society.
At the time of writing – May 2022 – there are still 33 issues outstanding. They have been open for months and there is no sign that any of these is being worked on. The presence of open issues is not in itself a problem. Most software is deployed with known issues, but these are always reported in the software release notes, along with descriptions of the appropriate mitigations. There do not appear to be any release notes for any version of this software.
One encouraging aspect of this repository is the presence of branches containing tests, as software is frequently built and launched without adequate testing. However, these are what we in the trade call ‘unit’ tests – used by developers to check their own work as it is written, compiled and built. There are no functional tests, and no high-level test plans nor any test strategy. There is no way for a disinterested tester to make any adequate assessment of the quality of this software. This is extremely poor practice.
This lack of testing resource is understandable when we realise that what we have in this GitHub is not the code that was used to produce Neil Ferguson’s “Report 9”: it is a reduced, reorganised and sanitised version of it. It appears that the state of the original 13,000-plus line monolith was so embarrassing that Ferguson’s financial backer, the Bill and Melinda Gates Foundation, rushed to save face by funding this GitHub (owned by Microsoft) and providing the expert resource necessary to carry out the conversion to something that at least looks like and performs like modern industrial software.
One side-effect of this conversion, though, has been the admission and indeed the highlighting, of a major problem that plagued the original system and made it so unstable as to be unsafe to use, although it was often denied.
We Knew It Was Wrong, Now We Know Why
One of the major controversies that almost immediately surrounded Neil Ferguson’s modelling software once the sanitised version of the code was released to the public gaze via GitHub was that it didn’t always produce the same results given the same inputs. In IT-speak, the code was said to be non-deterministic when it should have been deterministic. This was widely bruited as an example of poor code quality, and used as a reason why the simulations that resulted could not be trusted.
The Ferguson team replied with statements that the code was not intended to be deterministic, it was stochastic and was expected to produce different results every time.
Rather bizarrely, both sides of the argument were correct. The comments recorded as issues in GitHub explain why.
Before we go any further I need to explain a couple of computing terms – deterministic and stochastic.
Deterministic software will always provide the same outputs given the same inputs. This is what most people understand software to do – just provide a way of automating repetitive processes.
Stochastic software is used for statistical modelling and is designed to run many times, looping round with gradually changing parameters to calculate the evolution of a disease, or a population, or radioactive decay, or some other progressive process. This type of program is designed with randomised variables, either programmed at the start (exogenous) or altered on the fly by the results of actual runs (endogenous) and is intended to be run many times so that the results of many runs can be averaged after outlying results have been removed. Although detailed results will differ, all runs should provide results within a particular envelope that will be increasingly better defined as research progresses.
This is a well-known modelling technique, sometimes known as Monte Carlo simulation – I’ve programmed it myself many years ago, and have tested similar systems within ONS. Stochastic simulation can be very powerful, but the nature of the iterative process can compound small errors greatly, so it is difficult to get right. Testing this kind of program is a specialist matter, as outputs cannot be predicted beforehand.
To explain the initial problem fully: people who downloaded the code from GitHub and ran it more than once would find that the outputs from the simulation runs would be different every time, sometimes hugely so, with no apparent reason. Numbers of infections and deaths calculated could be different depending on the type of computer, operating system, amount of RAM, or even the time of day.
This led to a lot of immediate criticism that the software was non-deterministic. As mentioned above, the Ferguson team retorted that it was meant to be stochastic, not deterministic, and in the GitHub repository we find the following supporting notes (screenshot below). The third one is important.
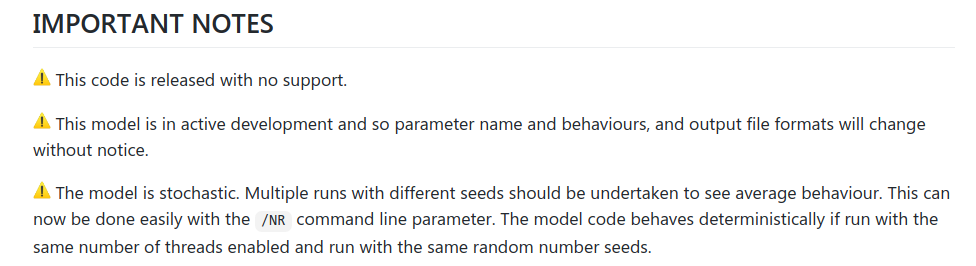
So it’s meant to be stochastic, not deterministic. That would be as expected for statistical modelling software. The problem is that, when we look at what the honest, upstanding engineers have written in their notes to themselves (screenshot below), we find this noted as Issue 161:
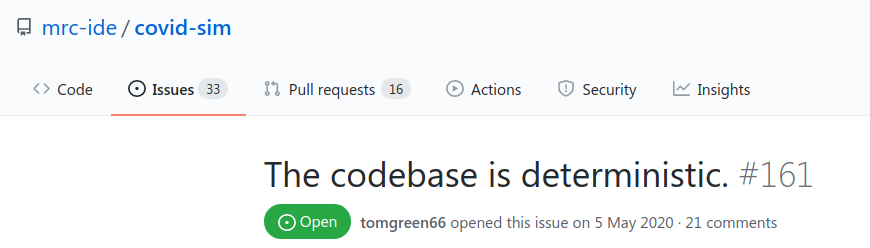
Of the 487 issues originally opened against this project, only one is considered important enough to be pinned to the top of the list. As you can see, it directly contradicts the assertions from the Ferguson team. That’s why engineers aren’t allowed to talk to customers or the public.
Reading the detail in Issue 161 reveals why the system is simultaneously deterministic and stochastic – a sort of Schrödinger’s model of mass destruction.
It turns out that the software essentially comprises two distinct parts: there is a setup (deterministic) stage that sets all of the exogenous variables and initialises data sets; and the second (stochastic) part that actually performs the projection runs and is the main point of the system.
The setup stage is intended to be – and in fact must be from a functional point of view – entirely deterministic. For the stochastic runs to be properly comparable, their initial exogenous variables must all be exactly the same every time the stochastic stage is invoked. Unfortunately, Issue 161 records that this was not the case, and explains why not.
It’s a bit technical, but it involves what we in the trade call ‘race conditions’. These can occur when several processes are run simultaneously by multi-threaded code and ‘race’ to their conclusions. Used properly, this technique can make programs run very quickly and efficiently by utilising all processor cores. Used wrongly, it can lead to unstable programs, and this is what has happened in this case. In the trade we would say that this software is not ‘thread safe’.
Race conditions happen when processes do not always take a consistently predicted amount of time to complete and terminate in unexpected sequences. There are many reasons why this may happen. This can mean that a new process can start before all of its own expected initial conditions are set correctly. In turn this makes the performance of the new process unpredictable, and this unpredictability can compound over perhaps many thousands of processes that will run before a program finishes. Where undetected race conditions occur, there may be many thousands of possible results, all apparently correct.
They may be due to differences in processor types or local libraries. There are many possible causes. Whatever the cause in this instance, every time this code was run before May 6th 2020 (and probably up to about 17th May 2020 when Issue 272 was opened and the code simplified) the result was unpredictable. That was why the results differed depending on where it was run and by whom, not because of its stochastic nature.
In itself, this problem is very bad and is the result of poor programming practice. It is now fixed so shouldn’t happen again. But, as we shall see, we cannot depend even on this new code for reliable outputs.
Where It All Came From and Why It Doesn’t Work
I have had the opportunity to work with a multitude of very capable professionals over the quarter century or so that I have been a freelance consultant, in many different spheres. Very capable in their own specialism, that is; when it came to writing code, they were nearly all disasters. Coding well is much harder than it seems. Despite what many people think, the development of software for high-consequence applications is best left not to subject experts, but to professional software developers.
I have no information as to the genesis of Professor Ferguson’s modelling code, but I’m willing to bet it followed a route similar to one that I have seen many times. Remember that this codebase first saw silicon many years ago, so came from a simpler, more innocent age.
- Clever Cookie (CC) has a difficult problem to solve
- CC has a new computer and a little bit of programming knowledge
- CC writes a program (eventually) to successfully solve a particular problem
- CC finds another problem similar to, but not identical to, the previous one
- CC hacks the existing code to cope with the new conditions
- CC modifies the program (eventually) to successfully solve the new problem
- GOTO 4
And so the cycle continues and the code grows over time. It may never stop growing until the CC retires or dies. Once either of those events happens, the code will probably also be retired, never to run again, because nobody else will understand it. Pet projects are very often matters of jealous secrecy and it is possible that no-one has ever even seen it run. There will be no documentation, no user guides, no designs, probably not even anything to say what it’s meant to do. There may be some internal comments in the code but they will no longer be reliable as they will have been written for an earlier iteration. There are almost certainly no earlier local versions of the code and it may only exist on a single computer. Worse than that, it may exist in several places, all subtly different in unknown ways. There would be no test reports to verify the quality of any of its manifestations and check for regression errors.
If Professor Ferguson had been suddenly removed from Imperial College in January 2020, I am confident that the situation described above would have obtained. The reason why I am confident about this is that none of the collateral that should accompany any development project has been lodged in the GitHub repository. Nothing at all. No designs, no functional or technical descriptions, no test strategy, test plans, test results, defect records or rectification reports; no release notes.
To all intents and purposes, there is no apparent meaningful design documentation and I know from talking with one of the contributors to the project that this is indeed the case. Despite this, Wes Hinsley and his team have managed to convert the original code (still never seen in public) into something that looks and behaves like industrial-quality bespoke software. How could have they managed to do this?
Well, they did have the undoubted advantage of easy access to the originator, ‘designer’ and developer of the software himself, Professor Neil Ferguson. In fact, given the rapid pace of the initial development, I suspect he was on hand pretty much all of the time. However, development would not have followed anything vaguely recognisable as sound industrial practice. How could it, with no design or test documentation?
I have been in this position several times, when clients have asked me to test software that replaces an existing system. On asking for the functional specifications, I am usually told that they don’t exist but “the developers have used the existing system, just check the outputs against that”. At that point I ask for the test reports for the existing system. When told that they don’t exist, I explain that there is no point in using a gauge of unknown quality and the conversation ends, sometimes in an embarrassed silence. I then have to spend a lot of time working out what the developers thought they were doing and generating specifications that can be agreed and tested.
What follows is only my surmise, but it’s one based on years of experience. I think that Wes was asked to help rescue Neil’s reputation in order to justify Boris’s capitulation to Neil’s apocalyptic prognostications. Neil couldn’t produce any designs so he and Wes (and the team) built some software using 21st century methods that would replicate the software that had produced the “Report 9” outputs.
As the remarkably swift development progressed, constant checks would be made against the original system, either comparing outputs against previous results or based on concurrent running with similar input parameters. The main part of the development appears to have been largely complete by the third week of June 2020.
The reason that I describe this as remarkably swift is that it is a complicated system with many inputs and variables, and for the first six weeks or so it would not have been at all possible to test it with any confidence at all in its outputs. In fact, for probably the first two months or so the outputs would probably have have been so variable as to be entirely meaningless.
Remember that it wasn’t until May 5th 2020 – six weeks after this conversion project started – that it was realised that the first (setup) part of the code didn’t work correctly at all so the second (iterative) part simply could not be relied on. Rectifying the first part would at least allow that part to be reliably tested, and although there is no evidence of this having actually happened, we can probably have some confidence that the setup routine produced reasonably consistent sets of exogenous variables from about May 12th 2020 onwards.
A stable setup routine would at least mean that the stochastic part of the software would start from the same point every time and its outputs should have been more consistent. Consistency across multiple runs, though, is no guarantee of quality in itself and the only external gauge would likely have been Professor Ferguson’s own estimations of their accuracy.
However, his own estimations will have been conditioned by his long experience with the original code and we cannot have much confidence in that, especially given its published outputs in every national or international emergency since Britain’s foot and mouth outbreak in 2001. These were nearly always wrong by one or two orders of magnitude (factors of 10 to 100 times). If the new system produced much the same outputs as the old system, it was also almost certainly wrong but we will probably never know, because that original code is still secret.
The IT industry gets away with a lack of rigour that would kill almost any other industry while at the same time often exerting influence far beyond its worth. This is because most people do not understand its voodoo and will simply believe the summations of plausible practitioners in preference to questioning them and perhaps appearing ignorant. Politicians do not enjoy appearing ignorant, however ignorant they may be.
In the next section we are going to go into some detail about the deficiencies of amateur code development and the difficulties of testing stochastic software. It will explain why clever people nearly always write bad software and why testing stochastic software is so hard to do that very few people do it properly. It’s a bit geeky so if you are not a real nerd, please feel free to skip on to the next section.
Gentlemen and Players
You’ll remember from above that our Clever Cookie (CC) had decided to automate some tricky problems by writing some clever code, using this procedure.
- CC has a difficult problem to solve
- CC has a new computer and a little bit of programming knowledge
- CC writes a program (eventually) to successfully solve a particular problem
- CC finds another problem similar to, but not identical to, the previous one
- CC hacks the existing code to cope with the new conditions
- CC modifies the program (eventually) to successfully solve the new problem
- GOTO 4
The very first code may well have been written at the kitchen table – it often is – and brought into work as a semi-complete prototype. More time is spent on it and eventually something that everyone agrees is useful is produced. The dominant development process is trial and error – lots of both – but something will run and produce an output.
For the first project there may well be some checking of the outputs against expected values and, as long as these match often enough and closely enough, the code will be pronounced a success and placed into production use.
When the second project comes along, the code will be hacked (an honourable term amongst developers) to serve its new purposes. The same trial and error will eventually yield a working system and it will be placed into production for the second purpose. It will also probably remain in production for its original purpose as well.
Now, during the development of the second project it is possible, although unlikely, that the outputs relevant to the first project are maintained. This would be done by running the same test routines as during the first development alongside the test routines for the second. When the third project comes along, the first and second test suites should be run alongside the new ones for the third.
This is called ‘regression testing’ and continues as long as any part of the code is under any kind of active development. Its purpose is to ensure that changes or additions to any part of the code do not adversely effect any existing part. Amateurs very rarely, if ever, perform this kind of testing and may not even understand the necessity for it.
If an error creeps into any part of the code that is shared between projects, that error will cascade into all affected projects and, if regression testing is not done, will exist in the codebase invisibly. As more and more projects are added, the effects of the errors may spread.
The very worst possibility is that the errors in the early projects are small, but grow slowly over time as code is added. If this happens (and it can!) the wrong results will not be noticed because there is no external gauge, and the outputs may drift very far from the correct values over time.
And this is just for deterministic software, the kind where you can predict exactly what should come out as long as you know what you put in. That is relatively easy to test, and yet hardly any amateur ever does it. With stochastic software, by definition and design, the outputs cannot be predicted. Is it even possible to test that?
Well, yes it is, but it is far from easy, and ultimately the quality of the software is a matter of judgement and probability rather than proof. To get an idea of just how complicated it can be, read this article that describes one approach.
Professional testers struggle to test stochastic software adequately, amateurs almost always go by the ‘smell test’. If it smells right, it is right.
Unfortunately, it can smell right and still be quite wrong. Because of the repetitive nature of stochastic systems, small errors in endogenous variables can amplify exponentially with successive iterations. If a sufficient number of loops is not tested, these amplifications may well not be noticed, even by professionals.
I recall testing a system for ONS that projected changes in small populations. This was to be used for long term planning of education, medical, transport and utilities resources, amongst other things, over a period of 25 years. A very extensive testing programme was conducted and everything ‘looked right’. The testers were happy, the statisticians were happy, the project manager was happy.
However, shortly before the system was placed in production use, it was accidentally run to simulate 40 years instead of 25 and this produced a bizarre effect. It was discovered that the number of dead people in the area (used to calculate cemetery and crematoria capacities) was reducing. Corpses were actually being resurrected!
The fault, of course, was initially tiny, but the repeated calculations amplified the changes in the endogenous variables exponentially and eventually the effect became obvious. This was in software that had been specified by expert statisticians, designed and developed by professional creators, and tested by me. What chance would amateurs have?
There are ways to test stochastic processes in deterministic ways but these work on similes of the original code, not the code itself. These are also extremely long-winded and expensive to conduct. By definition, stochastic software can only be approved based on a level of confidence, not an empirical proof of fitness for purpose.
Stochastic processes should only be used by people who have a deep understanding of how a process works, and this understanding may only be gained by osmosis from actually running the process. Although the projection depends on randomicity, that randomicity must be constrained and programmed, thus rendering it non-random. The success or failure of the projection is down to the choice of the variables used in setting the amount of random effect allowed in the calculation – too much and the final output envelope is huge and encompasses practically every possible value, too little and the calculation may as well be deterministic.
Because of the difficulties in testing stochastic software, eventually it always turns out that placing it into production use is an act of faith. In the case in point, that faith has been badly misplaced.
Mony a Mickle Maks a Muckle
Testing stochastic software is devilishly difficult. The paradoxical thing about that is that the calculations involved in most stochastic systems tend to be relatively simple.
The difficulties arise with the inherent assumptions and the definitions of the variables implicated in the calculations. Because the processing very often involves exponential calculations (we’ve heard a lot about exponential growth over the past 24 months), any very slightly excessive exponent can quickly amplify any effect beyond the limits of credibility.
To explain how exponential growth works, here are .01, .02, .05 and 0.10 exponential growths over 25 cycles. So for .05 growth per cycle each number is increased to the power 1.05, i.e., x = x1.05. The actual ‘exponent’ is the little number in superscript.

You can see from this table what an enormous difference a very small change in exponent can make – changing from 0.01 to 0.10 growth leads to a multiplying factor of about 900 over 25 cycles.
Now imagine that these results are being used as exponents in other calculations within the same cycles and the scale of the problem quickly becomes apparent. The choice of exponent values is down to the researcher and accurately reflects their own biases in the understanding of the system behaviour. In the case of infections, an optimist may assume that not many people will be infected from a casual encounter whereas a pessimist could see an entire city infected from a single cough.
This illustrates what a huge difference a very small mistake of judgement can produce. If we had been considering the possibility of a Covid-infected person passing that infection on to another person within any week was about 10%, then by the end of six months he would have infected over 1,800 people Each of those 1,800 would also have infected 920 others, and those 920 would have infected 500, and so on and so on.
However, if the real infection rate was just 1%, then the number of people affected after six months would probably not be more than a large handful. (This is massively over-simplified and ignores things like effectiveness of treatments, seasonality, differing strains and many more factors. But then, apparently, so does Neil Ferguson’s software.)
Many of the factors implicated in such calculations simply cannot be known at the outset, and researchers may have a valid reason to err on the side of caution. But in normal circumstances a range of scenarios covering the most optimistic, most probable and most pessimistic states would be run, with confidence levels reported with each.
As time passes and real world data is gathered, the assumptions can be tuned against findings, and the model should become more accurate and better reflective of the real world. Unfortunately, there is precious little evidence that Neil Ferguson ever considers the real world as anything other than incidental to his activities.
Remember that what we are considering is a code monolith – a single huge collection of programming statements that has been built up over many years. Given that the codebase is built up of C++, Erlang and R routines, its development may well have begun at the tail end of last century. It is extremely likely that its oldest routines formed the code that was used to predict the expected outcome of the 2001 outbreak of foot and mouth disease in the U.K. and it has been steadily growing ever since.
To gauge just how good its predictive capabilities are, here are some notable mortality predictions produced using versions of this software compared to their real-world outcomes. (An order of magnitude is a factor of 10 i.e., 100 is one order of magnitude greater than 10.)
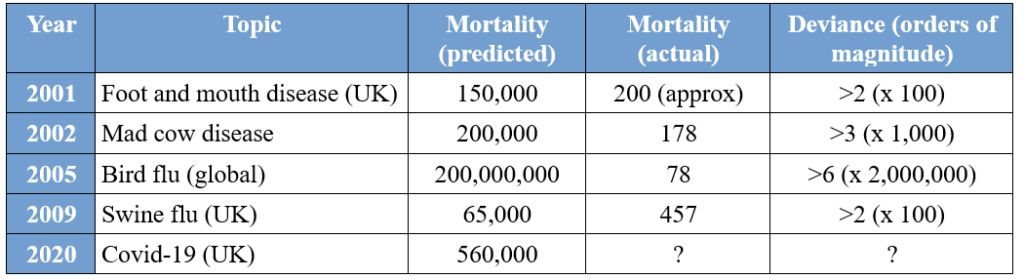
As we can see, the models have been hopelessly pessimistic for more than two decades. We can also see that, if any review of the model assumptions had ever been carried out in light of its poor predictive performance, any corrections made haven’t worked.
It appears that the greater the expected disaster, the less accurate is the prediction. For the 2005 bird flu outbreak (which saw a single infected wild bird in the U.K.) the prediction is wrong by a factor of over 2,000,000. It’s difficult to see how anyone could be more wrong than that.
I haven’t included a number for COVID-19 mortality there because it has become such a contentious issue that it is very difficult to report or estimate it with any confidence. A widely-reported number is 170,000, but this appears to be for all deaths of/with/from/vaguely related to COVID-19 since January 2020. In 2020 in England and Wales there were about 60,000 more deaths than in 2019, but there were only 18,157 notifications to PHE of COVID-19 cases, some, many or most of whom would have recovered.
As a data professional, it has been heartbreaking to watch the perversion, indeed the subversion, of data collection processes over the past two years. Definitions and methods were changed, standards were lowered and even financial inducements apparently offered for particular classifications of deaths.
Don’t Look Back in Anger – Don’t Look Back at All
I noted above that there seemed to have been precious little feedback from the real world back into the modelling process. This is obvious because over the period since the beginning of this panic there has been no attenuation of the severity of the warnings arising from the modelling – they have remained just as apocalyptic.
One of the aspects of the modelling that has been slightly ignored is that the models attempted to measure the course of the viral progress in the face of differing levels of mitigations, or ‘non-pharmaceutical interventions’ (NPIs). These are the wearing of face masks, the closure of places where people would mix, quarantines, working from home, etc. In total there were about two dozen different interventions that were considered, all modelled with a range of effectiveness.
The real world has stubbornly resisted collapsing in the ways that the models apparently thought it would, and this resilience is in the face of a population that has not behaved in the way that the modellers expected or the politicians commanded. Feeding accurate metrics of popular behaviour back into the Covid models would have shown that the effects expected by the models were badly exaggerated. Given that most people ignored some or all of the rules and yet we haven’t all died strongly suggests that the dangers of the virus embodied in the models are vastly over-emphasised, as are any effects of the NPIs.
On top of this we have the problem that the ways in which data was collected, collated and curated also changed markedly during the first quarter of 2020. These changes include loosening the requirements for certification of deaths, removing the need for autopsies or inquests in many cases, and the redefining of the word ‘case’ itself to mean a positive test result rather than an ill person.
At the start of the panic, the death of anyone who had ever been recorded as having had Covid was marked as a ‘Covid death’. This did indeed include the theoretical case of death under a bus long after successful recovery from the illness. Once this had been widely publicised, a time limit was imposed, but this was initially set at 60 days, long after patients had generally recovered. When even the 60-day limit was considered too long it was reduced to 28 days, more than double the time thought necessary to self-quarantine to protect the wider population.
There are other problems associated with the reporting of mortality data. We noted before that only 18,157 people had been notified as having a Covid diagnosis in England and Wales in 2020, yet the number of deaths reported by the government by the end of the year was about 130,000. It seems that this includes deaths where Covid was ‘mentioned’ on the death certificate, where Covid was never detected in life but found in a post mortem test, where the deceased was in a ward with Covid patients, or where someone thought that it ‘must have been’ due to Covid.
Given the amount and ferocity of the publicity surrounding this illness, it would be more than surprising if some medical staff were not simply pre-disposed to ascribe any death to this new plague just by subliminal suggestion. There will also be cases where laziness or ignorance will have led to this cause of death being recorded. If, as has been severally reported, financial inducements were sometimes offered for Covid diagnoses, it would be very surprising if this did not result in many more deaths being recorded as Covid than actually occurred.
Conclusions
Stochastic modelling is traditionally used to model a series of possible outcomes to inform professional judgement to choose an appropriate course of action. Projections produced using modelling are in no way any kind of ‘evidence’ – they are merely calculated conjecture to guide the already informed. Modelling is an investigative process and should never be done to explicitly support any specified course of action. What we have discovered, though, is that that is exactly how it has been used. Graham Medley, head of the SAGE modelling committee and yet another professor, confirmed that SAGE generally models “what we are asked to model”.
In other words, the scientists produce what the politicians ask for: they are not providing a range of scenarios along with estimates of which is most likely. Whilst the politicians were reassuring us that they had been ‘following the science’, they never admitted that they were actually directing the science they were following.
To demonstrate how different from reality the modelling may have been, we can simply examine some of the exogenous variables that are hard set in the code. In one place we find an R0 (reproduction) value of 2.2 (increasing quickly) being used in May 2020 when the real R0 was 0.7 to 0.9 (decreasing) and in March 2021 a value of 2 (increasing quickly) was being modelled when the real-world value was 0.8 to 1.1 (decreasing quickly to increasing slowly). Using these values would give much worse projections than the real world could experience. (As an aside, defining the same variable in more than one place is bad programming practice. Hard-coding is not good either.)
This admission has damaged the already poor reputation of modellers more than almost anything else over the past two years. We always knew that they weren’t very good, now it seems they were actively complicit in presenting a particular view of the disease and supporting a pre-determined political programme. Why they have prostituted their expertise in this manner is anyone’s guess but sycophancy, greed, lust for fame or just simple hubris are all plausible explanations.
One of the most serious criticisms of the Covid modelling is that it has only ever modelled one side of the equation, that of the direct effects of the virus. The consequential health and financial costs of the NPIs were never considered in any detail but in practice seem likely to hugely overshadow the direct losses from the disease. No private organisation would ever consider taking a course of action based on a partial and one-sided analysis of potential outcomes.
Wouldn’t it have been good if the software used to justify the devastation of our society had been subject to at least a modicum of disinterested oversight and quality assurance? Perhaps we need a clearing house to intervene between the modellers and the politicians and safeguard the interests of the people. It would surely be useful to have some independent numerate oversight of the advice that our legal or classically-trained politicians won’t understand anyway.
The best thing that can be said about the modelling that has been used to inform and support our Government’s response to the Covid virus is that is probably much better quality and of higher integrity than the nonsensical and sometimes blatantly fraudulent modelling that is ‘informing’ our climate change policy.
To join in with the discussion please make a donation to The Daily Sceptic.
Profanity and abuse will be removed and may lead to a permanent ban.