Citations are the currency of academia. Scholars who manage to gain a lot of them are more likely to get promoted, more likely to win prestigious awards and more likely to win the respect of their peers. An academic with a lot of citations is kind of like a City banker with a large end-of-year bonus – a big deal.
Given the importance attached to citation counts, scholars have an incentive to boost theirs by any means necessary. One practice that has received significant attention is self-citations. This is where individuals make a disproportionate number of citations to their own work. Another, related phenomenon is citation cartels. These refer to groups of individuals who agree to make a disproportionate number of citations to each other’s work.
Individuals who boost their citation counts through underhand practices obviously gain an unfair advantage over those who play by the rules. Hence there is considerable interest in detecting such practices, which are collectively termed ‘citation manipulation’.
In an interesting new paper, Hazem Ibrahim and colleagues investigate citation manipulation by analysing a dataset of 1.6 million Google scholar profiles. They find evidence that some individuals are indeed gaming the system. And they outline a method for identifying potentially suspicious individuals.
To look for evidence of citation manipulation, the authors began by filtering the dataset for individuals who saw large spikes in their citation counts in a particular year. They then zoomed-in further by identifying five individuals who received a large percentage of their citations from just a few papers that each cited them many times. These five individuals were deemed ‘suspicious’.
To check for evidence of citation manipulation, Ibrahim and colleagues compared the suspicious individuals to individuals who were similar in terms of field of research, year of first publication and total number of citations.
They began by computing the number of citations individuals in each group received in their peak citation year on Google Scholar and on Scopus (the latter only indexes journals that have been approved by an advisory board). They found that the matched individuals’ citation counts were only 45% lower on Scopus, whereas the suspicious individuals’ counts were 96% lower. This means that in the years when suspicious individuals saw large spikes in their citation counts, most of these citations came from journals not indexed by Scopus.
The authors proceeded to compute the number of citations individuals in each group received in the ten papers in which they were cited most. They found that the matched individuals were cited no more than 15 times in such papers, whereas the suspicious individuals were cited up to 45 times. This means that suspicious individuals received an excessive number of citations from a small number of papers.
Ibrahim and colleagues then propose a method for identifying potentially suspicious individuals, which involves computing two quantities for a certain individual.
The first is the ‘citation concentration’ or c2-index, defined as the largest number n such that there are n papers that each cite the individual at least n times. For example, if there are 10 papers that each cite the individual at least 10 times, they would have a c2 index of 10. The second quantity is the c2 percentage, defined as the percentage of the individual’s total citations that are accounted for by the n papers. For example, if 50% of the individual’s total citations are from the 10 papers, they would have a c2 percentage of 50.
The chart below shows the distribution of 900 individuals across dimensions corresponding to the two preceding quantities. The five suspicious individuals are highlighted in red. As you can see, most individuals are concentrated in the lower left-hand corner. By contrast, the five suspicious individuals are all located in the upper right-hand area. This discrepancy makes it very unlikely that all their citations are genuine.
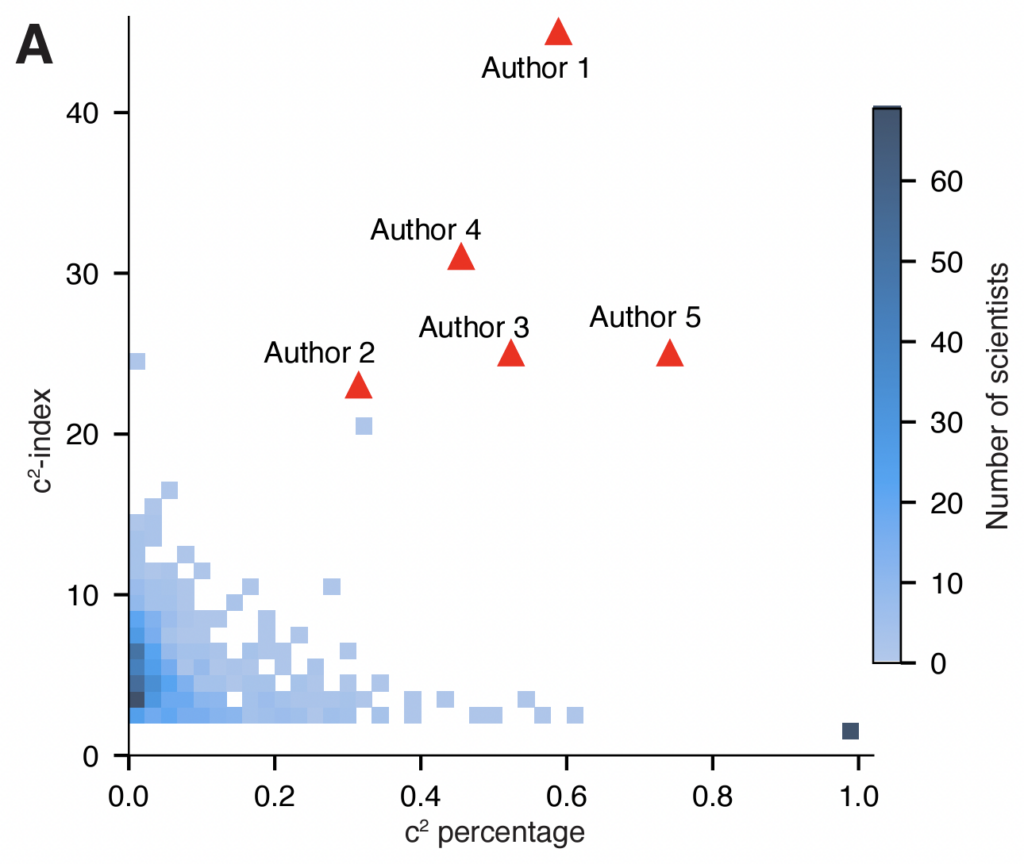
In the final part of their paper, the authors demonstrate that it is possible to boost one’s citation count on Google Scholar by creating AI-generated papers that include larger numbers of citations to one’s work and then posting those papers on preprint servers. They also demonstrate that it is possible to pay to have one’s citation count boosted: for a fee, fake journals will publish papers that include large numbers of citations to your work.
In terms of corrective actions, Ibrahim and colleagues call for bibliographic databases like Google Scholar to publish the c2-index alongside traditional citation metrics. Unfortunately, this is likely to be little more than a patch. If the c2-index does become widely published, bad actors could get around it by using AI to generate many papers that each cite the relevant individual a small number of times.
In an era of cheap and widely available artificial intelligence, stopping citation manipulation will be an uphill battle.
To join in with the discussion please make a donation to The Daily Sceptic.
Profanity and abuse will be removed and may lead to a permanent ban.
Israeli situation is discussed here.
https://www.rintrah.nl/suppression-of-the-innate-immune-system-the-main-cause-of-the-pandemic-of-the-fully-vaccinated/
We’re two months behind Israel.
Many thanks for posting that. It explains so much that is puzzling.
I thought of trying to summarize it, but no. It really does require reading in full. Just never get on the treadmill of these mRNA vaccines. (The Chinese may have got away with this, though the worry with their vaccines has always been ADE, so the jury is still out on that one.)
I think it’s rather telling – or rather, not telling – that we don’t distinguish between the experimental non-mRNA and the experimental mRNA pre-infection treatments when recording “vaccination” status along with critical care and deaths.
I’d love to see the breakdown in AZ versus Pfizer and Moderna.
This one I can corroborate – my sister-in-law, an ICU nurse, has reported empty, or nearly empty covid wards at the two hospitals she works at for several weeks. Given what most of us believe about the dangers of the ‘vaccines’ esp. OAS/ADE, wouldn’t we expect to see something more akin to Israel? How can we account for the difference? I’m baffled.
One difference would be that many-to-most UK citizens at high risk (i.e. the elderly) were dosed with Astra Zenica, the non-mRNA experimental treatment.
Apparently:
“Since more than half of ICU admissions have not been vaccinated, this suggests an intrinsically milder virus rather than just increased protection from vaccination.’”
According to the UKHSA there are around 5x more deaths in the vaccinated compared with the unvaccinated — now, that’s perhaps just because the vaccines are protecting them, but…
…Why aren’t these dying vaccinated people getting a bed in ICU? Are they dying before they get a chance to get admitted? Or maybe there’s a secret society of unvaccinated doctors turning the vaccinated away from ICU?
Or are these figures just really dodgy, as usual.
3 things spring to mind. Anecdotally, there seems to have been an upsurge in sudden deaths. These would appear to be predominantly among the vaccinated, so yes, dead before they get to ICU. Secondly, isn’t it possible that the treatment of patients may differ according to their vaccination status? If the unvaccinated are getting poorer treatment due to nhs staff bias, then maybe they’re more likely to deteriorate and need intensive care. Thirdly, I believe that ICNARC and UKHSA use different estimates for their unvaccinated population. ICNARC uses the ONS estimate, which likely underestimates how many people are unvaccinated, and therefore overestimates the proportion of unvaccinated that end up in ICU.
I have suspected for some time that treatment given in hospitals will differ according to jab status.
I’ve no proof, just a gut feeling as 20+ yrs experience of working with the medical profession means I am well aware of what some of them are capable of.
If everybody gets the same treatment why is one of the first questions about your jab status?
I agree. I can’t help but think there is something sinister, or at least very questionable, going on. A few weeks ago a relative (also a sceptic) told me he’d read an article in The Times which presented an anecdotal frontline report from an A&E doctor. In the report, reference was made (in passing) to having to identify the vaccine status of covid patients, as they were put in different areas “reflecting the need for different treatment”. What this meant in practice wasn’t explained.
The almost 5x was pure numbers, not proportion — in the last figures it was 5012 deaths with 2 or 3 doses, and 1177 unvaccinated.
I realised that. My comment about defining “unvaccinated “ denominators was just in response to your comment “or are these figures just really dodgy, as usual”. (If they can fudge something as basic as the uk population, what else will they fudge? Another one, clearly, is their definition of “unvaccinated”.) It wasn’t intended to explain the discrepancy per se. Perhaps I should have clarified at the time.
Yes these figures are definitely “dodgy”. I believe they don’t include admissions from care homes and have my suspicions that unvaccinated patients are rested much more regularly (and possibly at a higher cycle rate?) than the vaccinated. As you point out the vaccine shows completely different figures for deaths – around 80% jabbed. So the vaccine means that once hospitalised you are at a much higher risk of dying then? Or the figures are manipulated junk?
Spiegelhalter is a regime gatekeeper. Nothing he says can be taken at face value.
Israels problems may be related to the number who have been quadruple stabbed.
As the damage to the immune system is acknowledged to be cumulative, it may well be that the Israelis have many more over a threshold where their immune system can no longer cope?
This is just abuse of the term “vaccinated.”
Hospitalised but no injections – unvaccinated.
Hospitalised after one jab but exceeding the timeline for injection two = unvaccinated.
Hospitalised after two jabs, but not boosted to heaven, yet = unvaccinated.
I completely agree (see post). Add to this list “Unknown” which are also classed as “Unvaccinated”.
One can only suppose that this misinformation is being supported by doctors, just as they don’t report teenage heart attacks as adverse reactions (see Tommy Robinson’s Telegram posting yesterday – sorry, I can’t link to it) and continue to cash in on injecting while refusing to do their job of actually seeing patients.
The Australian tyranny is just a test run
https://www.conservativewoman.co.uk/the-australian-tyranny-is-just-a-test-run/
James Delingpole
Tuesday 8th February 2pm to 3pm
Yellow Boards By the Road
A329 junction London Road & Oak Avenue
Near Oakingham Belle pub
Wokingham RG40 1LH
Stand in the Park Sundays 10am make friends, ignore the madness & keep sane
Wokingham Howard Palmer Gardens Cockpit Path car park Sturges Rd RG40 2HD
Henley Mills Meadows (at the bandstand) Henley-on-Thames RG9 1DS
Telegram Group
http://t.me/astandintheparkbracknell
Delingpole’s article is superb – and the film is well worth watching (especially if they’ve sorted out the original volume problems)!
I think it is important that any time the term “Unvaccinated” is used it really needs to be in quotation marks since an unknown number of them have been vaccinated.
We need to show scorn and contempt for the way the term is used, as we should also for the term “Cases” and “Asymptomatic Infection”.
The German health and panic minister stated yesterday that Israel’s problems stem from too few elderly being vaxxed and boosted in comps with Europe.
As far as I can tell, that statement is completely fact free/a lie, it contradicts the statements from doctors there that 3/4 of the ICU patients are vaxxed and the general booster overload and lead there.
But even if it was true, it would then confirm that vaccinating people not at risk and younger ones was and is a misguided strategy and that any vaxx mandate for them, which he is now planning to introduce, is medically ridiculous.
Omicron seems to be having a much more significant impact on the population in both Israel and the USA.
Both of these countries used almost exclusively mRNA vaccines. The majority of adults in the UK were vaccinated with the Oxford Astra Zenica vaccine (at least the first two doses) Could it be that AZ is more effective at protecting from serious illness in the longer term than the mRNA vaccines?
It could be, yes. I’d note that while all the vaccines induce a pretty poor cellular immune response (but lots and lots of antibodies), AZ produced the highest amount of t-cell response (still poor, but higher than the others). We’ve little idea how any of the vaccines are modulating the innate immune response (there’s some papers saying that the mRNA vaccines at least have an impact on the innate immune system, but really we need much more data).
Alternatively, it could be a vaccine dose separation issue — in the UK we started vaccinating at the same time as Israel, but delayed the second dose by two months. This has put us two months behind Israel ever since.
It is called immune erosion. This is from the head of healthcare in Israel.
The Israelis have had 4 jabs. Their natural immunity has been more effectively suppressed (wrecked) than our population with a sizeable number who haven’t been jabbed, or have only had one or two.
Why “excluding Scotland”. Surely with vaxpässen and muzzle mandates still in force here, we must have reached zero by now? That’s what The Science demands, and reality must defer to it.
”One mystery is why Israel’s Omicron wave is proving a rougher ride in terms of ICU admissions and deaths compared to ours.”
Really? A ”mystery” is it? Not to a lot of us who’ve read about what jabs do to the immune system – and Israel has certainly done a lot of jabbing.
The Propaganda Loyal BBC & the Highly Trust worthy health & Prime Minister, with their Cameras & Photographers would have been all over this if they had a few patients. Lest not forget how much we spent on Nightingale Hospitals either!
Why is Israel’s situation as regards ‘COVID’ ICU numbers and deaths recently than here in the UK? Probably becuase:
1) They’ve used the Pfizer and Moderna vaccines throughout, which appear (in my view from reports) to cause immunity fatugue, i.e. their effectiveness wears off quicker and quicker and once it doesm the more vulnerable people’s immune systems are worse off.
They may be ‘dependent on the jabs’ so much that it could induce many auto-immune diseases, as some scientists and clinicians have speculated. Unfortunately, some many not know until years down the line, whereby it’s too late to make much of a difference.
The Oxford/AZ one did not seem to be anywhere near as ‘bad’ on that front, whilst lower initial immunity, it appears to last longer and not induce this immunity deficit so much/soon. Noticeably most oldies here got the AZ jab (my elderly parents did).
Whether this now changes in the coming months, as most of the booster jabs appear to be of the Pfizer/Moderna ones.
2) The has been a lower take up of jabs in the UK, especially in younger people, and also young children have not had them at all – yet.
Combine this and, in England (which makes up the majority of the UK population) lesser lockdown restrictions and more ignoring of those rules, the virus (especially since it ‘got milder’) has been far more extensively circulating in the below 50s population here, giving many more the better, longer-lasting natural immunity.
3) Israel rolled out the boosters quicker than we did, they are now on No. 4, but they rolled them out at a time of the year when they were least needed, and thus, IMHO, wasted before the aforementioned immune system problems kicked in just as the worst of winter illness was approaching.
It also means that the immune system problems are worsened because many have 2, not 1 booster and in quick succession.
What appears to be now coming out is that the effects of mRNA vaccines are still relatively unknown over the medium to longer term. To expect entire populations, inculding under 50s who in theory have decades of life to live, to have these jabs which are not fully tested despite them predominantly not being those seriously ill is an utter disgrace.
As more have said, any vaccines should have gone to just the most vulnerable, with them and everyone else using a holistic approach to both treatment and boosting the immune system by way of better diet, exercise/fresh air/sun, including vitmain D, zinc and a couple of other supplements, especially in colder less sunny months.
All the less vulnerable/younger people should then have just got on with their lives as before, shielding the vulnerable at the lowest level required without destroying what life they have left.
To show the Covid daily death figures are nonsense if the current trend continues we’ll shortly have fewer people seriously ill with Covid in ICU’s than die every day with Covid. A clear contradiction.
What “mystery” re Israel? The “vaccines” make many more susceptible to illness, as their immune systems get attacked by the snake oil. As per Mike Yeadon. mRNA mayhem is in the pipeline now…
Medical clinics and hospitals in USA are denying life-saving Ivermectin medicine even with court orders. Big Pharma doing all that they can to push the vaxx and inoculate us while effective and cheap COVID cures exist. There turns out to be censorship that we have never seen before for those who are looking for these treatments. We say over and over again that indepenedent researchers found Ivermectin safe and very effective for these Flu-Corona symptoms. Getting Ivermectin is easy https://ivmpharmacy.com