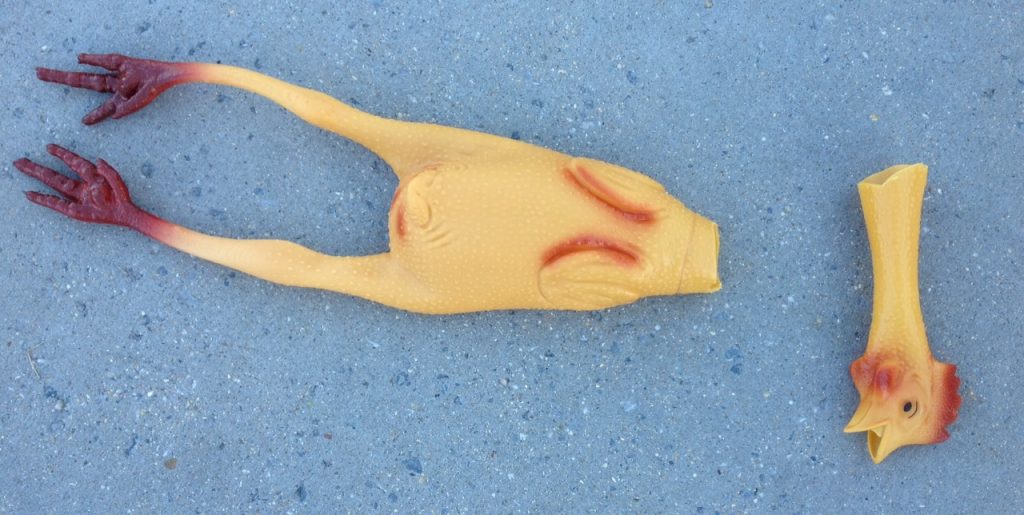
A man owned a chicken farm. One day he became concerned that it hadn’t rained for a while and that his crops, which he used to feed his chickens, might fail resulting in lots of chickens dying. So, the man went to the nearby temple to consult the Sage. After performing a complex ritual, the Sage had the answer: “You must sacrifice one of your chickens every week or it will never rain.” The man was upset – he liked his chickens and was always reluctant to kill them. But the Sage was wise and the ritual complex, so he obeyed the command and that evening he killed a chicken. The next day it rained.
We may well laugh at the man’s stupidity. How could he believe that sacrificing a chicken will have any relationship to the weather? But how do we prove that it doesn’t?
Clearly, the first time the man sacrificed a chicken it rained the next day, but this isn’t really evidence of cause and effect. So, rather than just this one case, what if we observed the man for a whole year? If we did this, we’d note that he sacrifices a chicken every week on a Tuesday night and that it rains in the subsequent week 10 times. From these observations we might conclude that chicken sacrifice has an efficacy of about 20% when it comes to making it rain. The problem with this is that because the man sacrifices a chicken every week there is a 100% certainty that he will have sacrificed a bird the week before it rains. So, this doesn’t help us as we are still unable to separate correlation from causality.
Thinking on this, it is clear we need to compare chicken killing with not chicken killing. Luckily his neighbour is a turnip farmer and does not sacrifice chickens and when we compare them, we see that it rains regardless of whether a chicken is killed or not. But what if turnip farming and chicken farming are not comparable when it comes to sacrifices and rainfall? To remove this as a possibility, we go and find lots of chicken farmers and randomly assign some of them to chicken sacrifice and others not. This way we are comparing like with like. Now we observe that there is no difference between these two groups with respect to whether it rains or not, and we’re really getting convinced that chicken killing is not making it rain. In the end we pull all of our observations together and publish them in CLUCK! (The magazine for Chicken Farmers) where we consider all of the evidence in the round and conclude firmly that there is no relationship between sacrificing chickens and whether it rains.
The points of this story are twofold. Firstly, it can be very difficult to disprove an assumed relationship between an intervention and an outcome once it is established as ‘truth’ and secondly, the only way we can do this is through building a case of ever higher quality evidence.
Putting aside poultricide, this kind of “hierarchy of evidence” is well established in clinical science. It recognises that not all data is equal, and that the strength of conclusions are different depending on the data used to underpin them. The following table summarises the different levels of clinical evidence together with how our chicken sacrificing story stacks up (adapted from Evans).
Strength of evidence | Type of evidence | Chicken Sacrifice |
Excellent | Systematic review multi-centre studies | The CLUCK! analysis of all of the evidence with respect to chicken sacrificed |
Good | Randomised controlled trials (RCTs) Observational studies | RCT with many chicken farmers |
Fair | Uncontrolled trials with dramatic (clear) results Before and after studies Non-randomised control trials | Comparing the chicken farmer to his turnip farmer neighbour |
Poor | Descriptive studies Case studies Export opinions Studies of poor methodological quality | The Sage’s advice The man killing a chicken and it raining the next day Describing the farmer’s chicken killing and rainfall patterns throughout the year |
This hierarchy is a useful tool in helping to understand how we might view the quality of evidence… especially when the evidence might be contradictory.
A couple of things to note. Firstly, ‘complexity of analysis’ is not a feature of this hierarchy. You might need to use complex analytical methods to understand some data sets, but simply using a complex approach doesn’t necessarily change where the evidence sits in this hierarchy: high quality analysis of poor-quality data still results in poor-quality evidence. The other thing to be aware of is that having many ‘poor’ pieces of evidence does not necessarily make the evidence any stronger: lots of experts having the same opinion does not mean that the opinion is automatically stronger evidence. We have to be very aware of things like confirmation bias when considering such multiple examples of ‘poor’ evidence.
Having established this hierarchy let’s have a look at lockdowns and COVID-19 through this lens.
Firstly, we need to decide on the question we’re addressing so that we can then see what the strength of evidence is that might help us answer it. In this case the question is simply “do lockdowns work?”, but we need to be a bit more specific about this and define “work” more explicitly:
Lockdowns work by avoiding more deaths due to COVID-19 than they cause due to other reasons.
As discussed by Ben Hawkins in his excellent piece “The Government is Gambling with People’s Lives”, lockdowns are a kind of Faustian ‘two-for-one’ pact where we agree to accept the deaths of some of our citizens to avoid the deaths or more. So, the evidence that we’re really interested in understanding is that which supports the numbers of deaths we are going to put into the Devil’s ledger.
My aim here is not to come up with specific numbers, but to think about the strength of the evidence that underpins them. Why is this important? Because in the debate about the effectiveness (or otherwise) of lockdowns we need to be sure that we are comparing like with like. For example, our chicken farmer might point to the fact that the Sage advised him to sacrifice chickens and when he does it sometimes rains as evidence that chicken sacrificing works. But this evidence is not of equivalent strength to the evidence showing there is no relationship between chicken sacrifice and rainfall. Both arguments deal with chickens, death and rainfall but they are not equally valid as the strength of evidence they are based on is not equivalent.
Back to lockdown
Let’s start by looking at the ‘debit’ side of things. Just as in our story with the chicken farmer, we need to have a comparison and in order to assess how many deaths will be caused by lockdown. In this case this is easy as we have lots of data gathered over many years as to what ‘no lockdown’ looks like with respect to deaths. In other words, we have an extensive ‘control’ set of data we can use to compare ‘no lockdown’ and ‘lockdown’. Also, because lockdowns demand imposition of societal restrictions (that is their ‘mode of action’ after all), then, unlike our chicken sacrifice/weather connection, we have clear cause and effect between some of these restrictions and resulting deaths. Overall, we are dealing with good quality, real world data and this will influence the strength of evidence used to draw conclusions about the number of deaths caused by lockdown.
However, most lockdown deaths will occur in the future and so are not explicitly ‘countable’ today. For example, we don’t actually know how a 30% reduction in breast cancer screening will be translated to an increase in women dying from this disease, just that it will. Because of this, the strength of evidence for any prediction of the total number of deaths due to lockdown is currently a matter of expert opinion with estimates varying between 100,000 to over 500,000. This means that at the moment, the precise number of deaths caused by lockdown is based on ‘poor’ strength of evidence. To be clear, this doesn’t mean that people are not going to die due to lockdown, just that we do not currently know how many. The reason for this is simply because most of these deaths haven’t happened yet but as time goes on the strength of the evidence will build as we count the corpses of those that lockdowns have taken.
Let’s move on to the ‘credit’ side of our ‘Faustian bargain’
What is the strength of evidence to support the number of COVID-19 deaths avoided by lockdown? We can easily count the number of COVID-19 related deaths that occur during lockdowns, but this doesn’t really answer the question as to how many we avoided. To do this we need a comparator, a ‘no lockdown’ control group. Unlike deaths due to lockdown where we had data from the UK prior to lockdowns for comparison, here we don’t have access to the data from a parallel universe where the UK had a COVID-19 pandemic but there was no lockdown. It is the need for such a control group that brings us to predictive computer modelling.
The evidence for the effectiveness of lockdowns in avoiding COVID-19 deaths is largely based on so called counterfactual ‘do nothing’ scenarios. These computer models themselves are very sophisticated things, but as we noted above, sophistication of analysis is not a definition of strength of evidence. Some computer models can be highly predictive (for example those used to help design cars or aircraft) but such models have been developed over years and their predictions rigorously validated with real world evidence. But these are not the types of models we are discussing here. So, how should we weight the strength of evidence from these ‘do nothing’ models?
These modelling predictions are expert opinions
Opinions supported by mathematics and computational science are beyond most of us (‘complex rituals’), but opinions all the same. Why? Because models codify the assumptions of the modellers who make them. So, for example, many ‘do nothing’ models are built on the dubious assumption that in response to a pandemic people will just carry on as if nothing is happening. In reality, of course, sick people change their behaviour and don’t need a dictatorial government edict to stay at home, or not to go to the pub, or not to go and see Nan. Where there is uncertainty (and there is uncertainty), then the modellers use their own judgement as to how to deal with this (see for example, Glen Bishop’s article on the lack of seasonality in Imperial College’s model, or this paper). Different models and different modellers = different opinions and different predictions. The strength of evidence for the effectiveness of lockdown based on such models is therefore ‘poor’.
A final point to remember is that these ‘do nothing’ models also generate predictions which are never tested. This is because, a bit like our chicken farmer sacrificing his chickens to avoid it never raining again, we always do something to avoid the predictions these ‘not doing something’ scenarios create. Remember when we killed all the cows because a model said there would be 500,000 deaths due to new variant CJD and there ended up being less than one hundredth of this number? Was the model a success or a failure? Did we actually avoid all these deaths or was the prediction just wrong? We can never know because we did something and didn’t get the result of doing nothing. We never tested the modelling prediction. As a result, these models are never validated, they are never proven to be remotely accurate, and so they will always be no more than just an opinion.
There is a fundamental philosophical question as to whether it is moral or ethical for a government to impose a policy which they know to kill people on the assumption that it might save more. But this is the one that lockdowns create. You might reasonably assume that given this seriousness, the decision to impose lockdown would only be taken if the evidence they were going to work was strong. However, the strength of evidence that lockdowns avoid more COVID-19 deaths than they cause is at the bottom of the hierarchy of evidence because it is based on unvalidated computer modelling predictions; we have imposed lockdowns on the population based on the opinions of a small number of experts. ‘Poor’ indeed.
Finally, I’m sure you want to know what happened to the chicken farmer. Well, he read the article in CLUCK!, which went through all of the evidence to show that sacrificing chickens did not cause it to rain, but he continued anyway. You see, he knew the Sage to be a very wise man and he was genuinely scared that if he stopped killing his chickens it might never rain again. In the end he ran out of chickens… and it still rained.
The author is a senior scientist working in clinical development.
Donate
We depend on your donations to keep this site going. Please give what you can.
Donate TodayComment on this Article
You’ll need to set up an account to comment if you don’t already have one. We ask for a minimum donation of £5 if you'd like to make a comment or post in our Forums.
Sign UpLatest News
Next PostCareful What We Wish For